ChatGPT as a Marketing Assistant
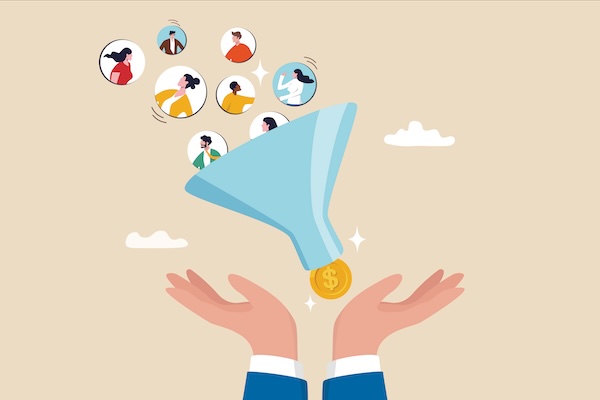
ChatGPT as a Marketing Assistant
October 16 | By Professor Professor Paul Ellickson
In this blog post, Professor Paul Ellickson describes the potential of machine learning and Generative AI tools to predict and improve the performance of marketing campaigns.
Identifying and optimizing targeted promotional strategies is a critical challenge for marketing professionals and researchers alike. For marketing managers, it’s essential to evaluate how current promotions perform so that future campaigns are better tailored to the firm’s customers.
Forecasting the success of a promotional campaign is a difficult task for any marketing team. For example, the use of email marketing, where both the subject line and the body of the email can be tailored for response, can create an endless number of campaigns, each resulting in varying levels of engagement from the customers. With the advent of techniques in causal inference, machine learning, and Generative AI, marketing decisions can move away from the use of heuristics to data-driven content generation and targeting.
The key to this transition lies in the ability of the manager to leverage the above techniques to better evaluate the heterogenous responses of customers to heterogenous interventions. Once such responses are estimated, managers can engage in effective targeting by matching the correct content with the customer who reacts to it most favorably. Below, I summarize the results of two research papers that show how causal inference, machine learning, and Generative AI can work in tandem to help marketing professionals understand the effect of their promotional choices on outcomes and improve content generation.
Laying the Groundwork
Most marketing studies focus on the effects of binary interventions. An example of this is studying the changes in a customer’s purchasing habits after receiving an email. Yet, this aggregation of interventions may obfuscate more nuanced patterns in the data. In a 2023 paper, Estimating Marketing Component Effects: Double Machine Learning from Targeted Digital Promotions, my co-authors and I explore how different marketing campaigns perform based on their differing content. We examine 34 email promotions sent by a women’s clothing company to 1.3 million customers over a 45-day period to determine the effectiveness of different subject lines. Other than one-offs like a “Happy Birthday” email, most of the subject lines referenced promotions like a holiday sale, specific item on sale, or shipping discount.
In our paper, we use machine learning techniques to create a framework that measures the impact of these different subject lines by leveraging and extending advances in causal inference.
Here are several of our key findings:
- Granular Component Analysis: Traditional practice often categorizes promotions into binary groups, such as price promotions and non-price promotions. Our results reveal that the effectiveness of a promotion is frequently driven by the specific marketing components within it. For example, instead of treating “Free Shipping and Free Returns” as a single non-price promotion, marketing managers would benefit from separately measuring the effects of “Free Shipping” and “Free Returns.”
- Content Performance Insights: Separating out semantic elements from promotional ones, we show that these costless enhancements drive further engagement. For example, semantic enhancements such as “Exclusive 40% Off” versus simply “40% Off” increase conversion rates. Further, personalized content, like “Happy Birthday” emails, drives even greater engagement.
- Targeting Strategies: The retailer sends better offers to less engaged customers. For instance, a customer who recently made a purchase might receive an email offering 30% off their next purchase, while someone who hasn’t purchased in a while might receive a 40% off promotion. Although the 40% promotion appears less effective at first glance, after adjusting for customer engagement levels, it becomes clear that the larger promotion yields a greater response and that the firm would be better off reversing its targeting strategy.
- These insights give managers information to better target customers by level of engagement. We estimate that by leveraging our framework, the company could increase profitability by approximately 11%.
Introducing ChatGPT
This year, my co-authors and I released a second paper (Using Contextual Embeddings to Predict the Effectiveness of Novel Heterogeneous Treatments) that is aimed at explaining the relative performance of the emails we examined in the first paper.
To allow direct estimation of observable effects, our prior work focused on operationalizing the observable set of characteristics. Yet, it is likely these characteristics do not function in isolation. Instead, the placement of promotions and semantic choices together create a synergistic utility for the customer. Being able to directly catalog such information was a previously intractable problem. In recent years, large language models (LLMs) have made it possible to convert text into vectors of numbers called contextual embeddings. Surprisingly, a relatively short numeric vector can convey the meaning of long, complicated paragraph of text. We use these contextual embedding to create a latent causal framework that allows for both estimation of causal effects and, most importantly, forecasting of new creatives not seen within the data. As a test, we predict the performance of hypothetical emails that can be compared to the existing 34 email promotions we included in our analysis. Obtaining the set of contextual embeddings, we present ChatGPT with the 34 emails. Further, we ask ChatGPT to act as an ideation engine to develop new content based on the observed set of emails. Our latent class causal framework gives us the ability to evaluate the performance of those new emails.
Here are our findings:
- The framework we created is 78.6% effective in explaining the performance of each email compared to the rest.
- Our framework is 65% accurate in predicting the monetary performance of each email—i.e., how much spend results from the email.
- One of the emails ChatGPT suggests performs well compared to the rest of the emails, but the other four do not.
The Bottom Line
When it comes to augmenting the efforts of design and marketing professionals, ChatGPT lives up to the hype. It might not sound impressive for it to come up with one high-performing email title and four low-performing ones, but 20% is enough to revolutionize a company’s marketing practice at scale. We only asked ChatGPT to come up with five ideas for emails that will engage customers. If it came up with 200 ideas and 40 were high-performing (according to our predictions), that is enough to create an email campaign larger than the one we studied. Even if a team is not comfortable with using ChatGPT’s recommendations to form the bulk of a campaign, ChatGPT can serve as an engine for pre-processing ideas.
Our research demonstrates that the use of latent class causal analysis combined with Generative AI has promise in both academic and managerial tasks. We focus on a simple setting of email marketing, as email subject lines are an easy use case to explore. However, we hope to go beyond subject lines to the actual email content to discover whether we can glean the same performance data. We have even considered evaluating embeddings from spoken words, like an ad in a podcast. As Generative AI becomes more sophisticated, there is no limit to the marketing applications we can explore.
Paul Ellickson is the Michael and Diane Jones Professor of Marketing and Economics at Simon Business School.
Follow the Dean’s Corner blog for more expert commentary on timely topics in business, economics, policy, and management education. To view other blogs in this series, visit the Dean's Corner Main Page.