AI and the future of work
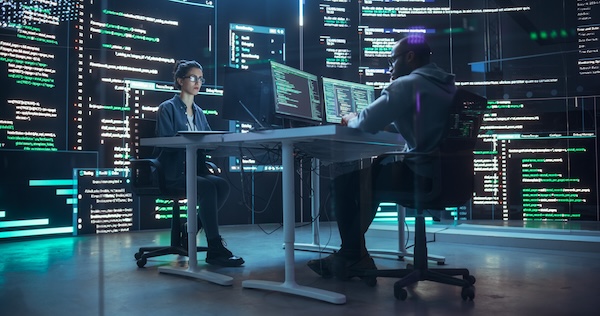
AI and the future of work
December 14, 2023 | By Professor Huaxia Rui
Last year, the advent of ChatGPT raised new questions about what Artificial Intelligence (AI) means for human labor. Workers who once felt secure in their jobs began wondering if they would soon go the way of the telegraph operator or the carriage driver.
In a working paper, my co-authors and I create a visual framework to think about the evolving relationship between AI and jobs. We then use the launch of ChatGPT as a shock to test an idea we call the inflection point conjecture.
A conceptual framework
Before diving in, let’s address three common misunderstandings.
First, intelligence is not the same as consciousness. While we can define human or artificial intelligence in various job contexts, the same cannot be said for consciousness. In fact, whether consciousness even exists remains debatable.
Second, there are really two forms of human intelligence: one based on deduction and the other based on induction, much like System 2 (slow thinking) and System 1 (fast thinking) suggested by psychologist Daniel Kahneman. We can think of deduction as a causal inference process based on logic and premises, and induction as a computational process of achieving generalization using data under certain distribution assumptions. Hence, we need to distinguish Statistical AI and Causal AI where the former, better known as machine learning, obtains knowledge by detecting statistical regularities in data. Statistical AI gained momentum later last century, thanks to significant progresses in statistical and computational learning theories, and, of course, to the dramatic increase in computing power and the availability of vast quantities of data. Current AI technologies are largely based on Statistical AI. Despite its limitations in reasoning, Statistical AI has enjoyed enormous success over the past decade or so. It most likely will be the form of AI that revolutionizes the way we live and work in the near future.
Third, current AI technologies are task-specific, not task-generic. Artificial general intelligence (AGI) that can learn any task is probably still decades away, although some have argued that GPT-4’s capabilities show some early signs of AGI.
We limit our discussions to task-specific Statistical AI and will refer to it as AI from now on.
The power of AI for a given task depends on four factors.
Task learnability—how difficult it is for an AI to learn to complete a task as well as a human worker does. From the perspective of an AI, a task is essentially a function mapping certain task input to some desirable task output, or more generally, distribution of task outputs. The learnability of the task is determined by how complex the mapping is and how difficult it is to learn this mapping from data using computational algorithms. While some tasks are highly learnable because they are so routine, others may require vast amounts of data and/or huge amounts of computational resources for the learning to be successful. In fact, there may even exist tasks that are simply not learnable no matter how much data we have. As a theoretical example, consider the practical impossibility of learning the private key in a public-key cryptosystem, even though one can generate an arbitrary number of labeled instances, i.e., pairs of plaintext and encrypted messages.
We can break down a task’s learnability into its statistical complexity Sf and its computational complexity Cf. Visually, we may represent a task as a point on a task plane where the two coordinates represent the statistical and computational complexities of the task. Plotting AI performance (e.g., relative to human performance) for all tasks in a 3-dimensional space which we refer to as the task intelligence space, we obtain the current intelligence surface, or CIS, for short, that represent the overall intelligence levels of current AI technologies. The top left panel of Figure 1 illustrates this concept.
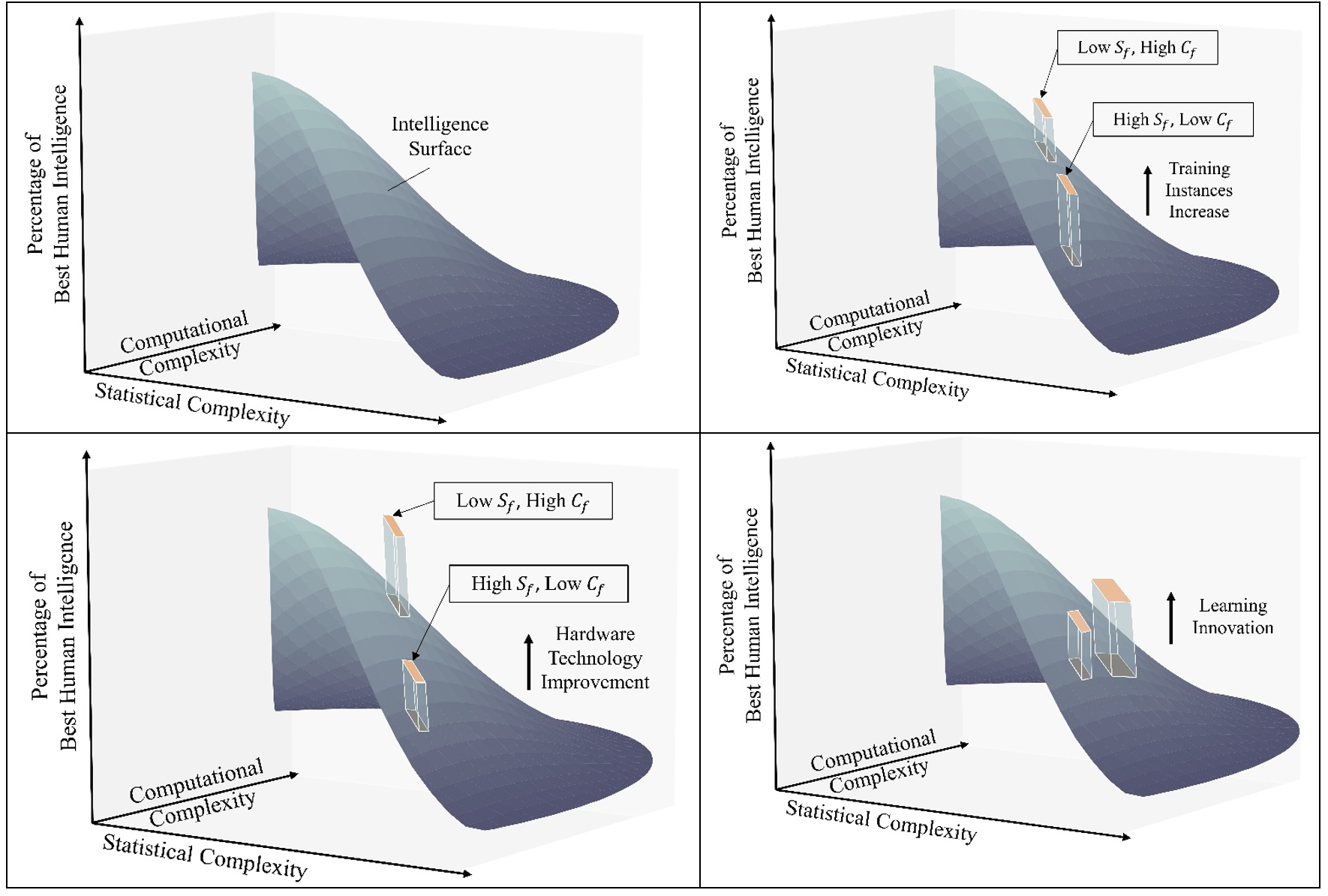
Figure 1
The two sources of task learnability imply two types of resources needed for AI to successfully learn the task, which lead us to the next two factors.
Data availability—The more data points available to train an AI, the higher the CIS is. Whether it is data about driving conditions and vehicle control to train an autonomous vehicle or documents in different languages to train a translation device, the availability of sufficient amounts of labeled data is of paramount importance for AI to approximate human intelligence. This may seem obvious given our understanding of the two types of resources required for the training of AI. Its significance in practice can still be strikingly impressive. For example, the ImageNet project, launched in 2009 and containing more than 14 million annotated images of over 20,000 categories, is of historical importance in the development of AI, especially for vision tasks. Dr. Fei-Fei Li, the founder of ImageNet, is recognized as the godmother of AI at least in part for establishing ImageNet. Because the importance of data availability for different tasks depends on their degrees of statistical complexity, as is illustrated in the top right panel of Figure 1, we may also understand the significance of ImageNet for vision tasks by noting the high statistical complexity of image data.
Computation speed—The faster the computation speed is, the higher the CIS is. Similarly, the importance of computation speed for different tasks depends on their degrees of computational complexity, as is shown in the bottom left panel of Figure 1. The recent example of graphic processing unit, or GPU, demonstrates the importance of this factor.
Learning techniques—Unlike the first factor, which is an inherent property of a task, or the second and third factors, which are resources, this factor is all about the actual learning and is where unexpected progress is made thanks to human ingenuity. It encompasses a variety of techniques used for learning which can be broadly categorized into two types: better hypothesis class or better learning algorithm. For example, the successes of convolutional neural networks for computer vision tasks and the transformer architecture for natural language processing are examples of better hypothesis classes. On the other hand, regularization and normalization techniques are examples of learning algorithm improvements. If there is an occupation that will never be replaced by task-specific Statistical AI, we bet on researchers and engineers who innovate in learning techniques. The bottom right panel of Figure 1 illustrates the impact of improvements in learning techniques, the magnitude of which is not necessarily related to task learnability.
In summary, we can understand the AI performance through the lens of four factors, illustrated in Figure 2.
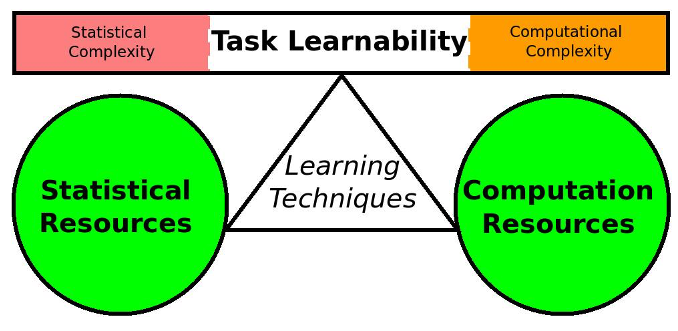
Figure 2
For a given task, whether AI performance is satisfactory enough depends on what we mean by satisfactory. To make this concrete, imagine another surface, referred to as the minimal intelligence surface, which represents the minimal level of AI performance for us humans to consider it as satisfactory. If the CIS is below the minimal intelligence surface on a task, AI performance on that task is not yet good enough and the task remains a human task. But if the CIS is above the minimal intelligence surface on a task, the task can be left for AI.
Three phases for AI-jobs relations
We consider an occupation as a set of tasks. Depending on the relative position of the CIS and the minimal intelligence surface, we can play out three different scenarios.
Phase 1: Decoupled
This is the phase when human workers are not engaging with AI while doing their jobs. Graphically, the CIS is below the minimal intelligence surface on the region corresponding to the task set of the occupation, as is illustrated in the left panel of Figure 3 where the occupation is represented by six red dots. Therefore, none of the tasks can be satisfactorily completed by AI yet. This phase will likely last long for occupations with data availability issues.
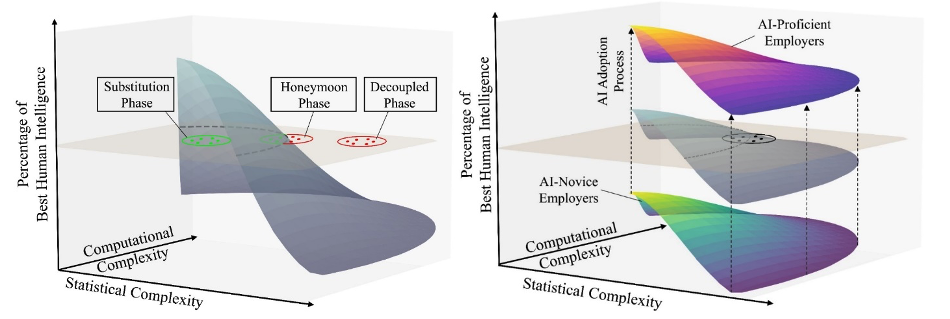
Figure 3
Phase 2: Honeymoon
This is the phase when human workers and AI benefit from each other. Graphically, the CIS is above the minimal intelligence surface on some tasks of an occupation but is below the minimal intelligence surface on other tasks of the occupation. In other words, these jobs still have to be done by human workers, but AI can help them by satisfactorily completing some of the tasks required by the jobs. On the other hand, by working side-by-side with human workers, the AI can benefit from new data generated by human workers. In the left panel of Figure 3, we illustrate this phase by representing the occupation using six dots. The three green dots represent the tasks that an AI can do, and the three red dots represent the tasks that only a human can do. Human workers of such an occupation will use AI to complement their work, benefiting from the boost in productivity that comes from offloading some tasks. Ironically, this may also accelerate their own replacement.
Phase 3: Substitution
In this phase, AI can perform as well as an average human worker but at a much smaller or even negligible marginal cost. Graphically, the CIS is completely above minimal intelligence surface on the region corresponding to the task set of the occupation. In the left panel of Figure 3, we illustrate this phase by representing the occupation using only green dots. At this phase, the occupation is at the risk of becoming obsolete because the marginal cost of AI is often negligible compared to that of humans, making it more efficient for these jobs to be completed by AI rather than by humans.
While the minimal intelligence surface is largely static, the CIS shifts upwards over time, because even though task learnability is an inherent task property, the other three factors progress over time, resulting in improved AI performance. Hence, we can envision most occupations, initially decoupled with AI, gradually enter the honeymoon phase, and for many, eventually move into the substitution phase. On the other hand, because AI adoption takes time and different organizations have different AI proficiency levels, we may find that the same occupation can simultaneously be in different phases, depending on organizations or regions. We illustrate this point in the right panel of Figure 3.
The Inflection Point
Based on the conceptual framework, we further build and analyze an economic model to show the existence of an inflection point for each occupation. Before AI performance crosses the inflection point, human workers always benefit from improvement in AI, but after the inflection point, human workers become worse off whenever AI gets better. This model insight offers a way to test our thinking using data. Let’s consider the occupation of translation and the occupation of web development. Existing evidence suggests that AI likely has crossed the inflection point for translation, but not for web development. Based on the inflection point conjecture, we hypothesized that the launch of ChatGPT has likely benefited web developers but hurt translators. We believe these effects should be discernible in data because the launch of ChatGPT by OpenAI a year ago significantly shocked the CIS, affecting many occupations. Indeed, anecdotal evidence and our own experiences suggest that ChatGPT has increased AI performance for translation and for programming in general. There are even academic discussions that ChatGPT, especially the one powered by GPT-4, has shown early signs of AGI which is the stated mission of OpenAI.
To test this, my co-authors and I conducted an empirical study to evaluate how the ChatGPT launch affected translators and web developers on a large online freelance platform. Consistent with our hypotheses, we find that translators are negatively affected by the launch in terms of the number of accepted jobs and the earnings from those jobs. In contrast, web developers are positively affected by the same shock.
By nature, some occupations will be slower to enter the substitution phase.
Occupations that require a high level of emotional intelligence will be slower to enter the substitution phase. At a daycare center, for example, machines may replace human caregivers by changing diapers and preparing bottles, but they will be poor at replicating human empathy and compassion. Humans are born with a neural network that can quickly learn to detect and react to human emotions. That learning probably began tens of millions of years ago and has become engrained in our hardware. Machines, in contrast, do not have that long evolutionary past, and must learn from scratch, if they can learn at all. At a more fundamental level, this might be rooted in the computational complexity of learning to “feel”.
Occupations that require unexpected or unusual thinking processes will also be slower to enter the substitution phase or even the honeymoon phase. Humans sometimes come up with original ideas seemingly out of nowhere, without following any pattern. What’s more intriguing is that we may not be able to explain how we came up with that idea. While fascinating for humans, this poses significant challenges to AI because there simply isn’t enough data to learn from. To exaggerate a bit, there is only one Mozart, not one Mozart a year.
What’s next
The relationship between AI and humans is already generating heated public debates because of its profound implications on our society and the potential to disrupt the fabric of our society. At this moment, I still believe there is a future for human workers, not only because of the many limitations of current AI technologies, but also because of our limited understanding of ourselves. Until the moment when we finally understand what it means to be human and the nature of human spark, we have a role to play in the cosmic drama.
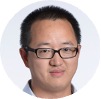
Huaxia Rui is the Xerox Professor of Information Systems at Simon Business School.
Follow the Dean’s Corner blog for more expert commentary on timely topics in business, economics, policy, and management education. To view other blogs in this series, visit the Dean's Corner Main Page.